The accelerated resolution of millions of cases from days to hours through AI
Accelerating Case Resolution from Days to Hours through AI
Driving profitability and growth for a support services organization by delivering better customer experiences, accelerating case resolution times, and providing additional features and services
The client, a Fortune 50 networking equipment manufacturer, runs one of the largest and most complex technical support organizations in the world. Their 10,000 engineers handle millions of cases annually for companies ranging from global service providers to small family businesses. Repeatedly recognized as an innovator in the space, the effectiveness and efficiency of this support organization has played a key role in company's growth over the past decade. Now, the organization is facing pressure on two sides. First, the complexity and number of products in the company's portfolio has grown exponentially over the years. The burden of supporting these new technologies means that cases are taking longer to troubleshoot, and that engineers are spending more time handling routine problems. Second, the competition for technical support is rapidly catching up, and customers are demanding faster and lower-cost services. In order to lay the foundations for the future success of the organization, the company's leadership team faced the challenge of developing and implementing an innovation strategy that could accelerate case resolution times from days to hours and scale across hundreds of new technologies.
THE APPROACH
A key part of the organization's innovation strategy is to build AI and cognitive computing capabilities to help automate the resolution of routine or repeated customer cases, so that engineers can focus their time on the most critical problems. One of the biggest challenges in resolving these routine cases is performing the initial diagnostics of the problem based on the customer's described symptoms; once the exact nature of the problem is identified, a standard action plan and set of troubleshooting steps can typically be provided to the customer to resolve the issue.Chobanian Group was brought in to help build an AI system to diagnose one of their hardest and most problematic technologies to troubleshoot: Virtual Private Networks. Virtual Private Networks involve multiple systems working together to provide functionality, which makes pinpointing problems especially difficult. If AI could be demonstrated to be effective here, the process could be replicated across other technologies in the company's portfolio.
THE SOLUTION
Chobanian Group first helped establish a repeatable machine learning pipeline using open-source tools, where different learning algorithms could systematically be evaluated while incorporating user input and feedback. Using this pipeline, the team was able to iteratively refine the initial models into a deployment-ready model that provided over 65% accuracy in diagnosing a number of VPN problems – something that is difficult even for human engineers with years of experience. CG then helped the team develop a machine learning platform to deploy the model. The platform provided an open API that the organization's case management system and other downstream applications could use to perform real-time diagnostics of new VPN support cases. It was also designed to be self-optimizing, so engineers could add new training data or correct mistakes to improve the model's performance over time. Every night, the platform automatically performed a model shoot-out, testing dozens of different algorithms revealing the best performing model for the current data.
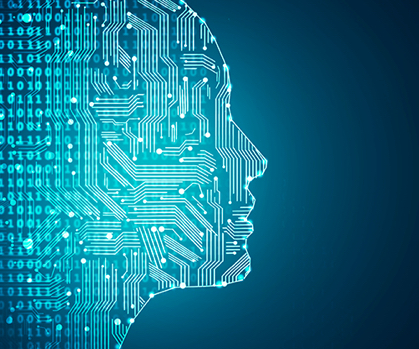
THE RESULTS
The machine learning platform enabled the team to rapidly launch a successful proof-of-concept program, using the organization's existing case management system, to demonstrate the effectiveness of the model on new live incoming VPN cases. The results of this POC program provided immediate value to support teams, and gave clarity on how to build an effective AI system that was fast, low cost and effective – saving multiple fiscal quarters of development time and hundreds of thousands of dollars in R&D.For more information, contact us at: hello@chobaniangroup.com